As enterprises accelerate their digital transformation journeys, more and more organizations now rank automation as a top strategic priority. Yet, amidst the race to streamline operations and cut costs, business leaders face a critical dilemma: Should they invest in Robotic Process Automation (RPA) for its task-specific efficiency, or pivot to AI Agents capable of adaptive decision-making?
This article cuts through the hype to deliver a pragmatic comparison of RPA and AI Agent technologies. We’ll analyze their distinct capabilities through real-world use cases, reveal how forward-thinking enterprises are blending both tools via hyperautomation frameworks, and provide a concrete decision matrix to align your choice with specific operational needs.
Whether you’re optimizing supply chain logistics or reimagining customer engagement, understanding these technologies’ synergies and limitations will prove vital to building a future-ready automation roadmap.
Understanding Core Concepts
What is RPA?
Robotic Process Automation (RPA) functions as a digital workforce designed to replicate human interactions with software systems. Unlike traditional automation tools, RPA bots operate at the user interface level, mimicking how employees navigate through applications like ERP systems or legacy databases.
This technology excels in environments requiring strict adherence to predefined rules, such as processing standardized invoices, migrating structured data between platforms, or executing compliance-heavy financial reconciliations. Its strength lies in handling high-volume repetitive tasks with clockwork precision – think of it as a tireless virtual clerk that never mis-clicks or needs coffee breaks. However, RPA’s limitation becomes apparent when faced with unstructured data or decision-making scenarios, as it lacks cognitive capabilities to interpret context or adapt workflows autonomously.
What is an AI Agent?
An AI Agent represents the next evolutionary step in business automation, combining machine learning, natural language processing (NLP), and adaptive reasoning to emulate human-like decision-making. Unlike its rule-bound RPA counterpart, an AI Agent thrives in ambiguous environments – it can analyze customer sentiment from messy email threads, predict inventory needs using disparate data sources, or resolve complex service tickets by understanding intent behind poorly phrased requests.
These systems learn and improve over time through continuous feedback loops, enabling capabilities like a conversational commerce chatbot that personalizes recommendations based on real-time browsing behavior. While requiring more substantial initial investment in training data and model development, AI Agents unlock value in processes where variability and judgment traditionally demanded human intervention, effectively serving as cognitive partners rather than mere task executors.
Key Differences Between RPA and AI Agent
Currently, the topic of AI agent is very hot, so what is the difference between AI agent and RPA, AI agent can replace RPA? McKinsey also points out that companies need to catch this AI Agent trend.
AI Agent (Artificial Intelligence Agent) and RPA (Robotic Process Automation, Robotic Process Automation) are both automation technologies, but there are some differences between them in terms of their functions, scope of application and working principles:
RPA | AI Agents | |
Technology Basis | Rule-based automation using predefined scripts and conditional logic | Advanced technology leveraging machine learning to mimic intelligent behavior |
Autonomy | Requires manual rule setting, limited to predefined processes | Capable of self-learning, autonomous improvement, and adaptive decision-making |
Application Scope | Ideal for structured, repetitive tasks like:Data entry, Report generation, Simple transaction processing | Versatile applications:customer service;complex data analysis;predictive insights;dynamic problem-solving |
Degree of Intelligence | A relatively low level of intelligence and mainly performs predefined tasks | Higher intelligence and is able to understand and process complex tasks such as natural language and image recognition |
Adaptability | Is less adaptable to environmental changes and needs to be manually adjusted when processes or rules change | AI agents can better adapt to environmental changes and even predict and adapt to unknown situations |
Advantages of RPA over AI Agents
- Determinism and predictability: RPA is very predictable in its behaviors and results because it adheres to clear, predetermined rules. Because AI agents are probabilistic, their behavior can be more unpredictable.
- Reduced implementation complexity: RPA can be set up by business users with little training and often requires less specialized technical knowledge to implement.
- Reduced start-up costs: RPA systems typically prove more affordable to implement than AI solutions, which demand huge investments in data, infrastructure, and specialist manpower.
- Transparency and auditability: RPA processes are easy to audit and transparent, which is important in highly regulated fields like healthcare and finance.
Adaptability of RPA and AI Agent
RPA has a relatively low level of intelligence and mainly performs predefined tasks, while AI Agent has higher intelligence and is able to understand and process complex tasks such as natural language and image recognition.
Process change
RPA is less adaptable to environmental changes and needs to be manually adjusted when processes or rules change. AI agents can better adapt to environmental changes and even predict and adapt to unknown situations.
Task difficulty
Regarding whether AI Agent can replace RPA, it depends on the specific situation:
In some simple automated tasks with clear rules, RPA may still be the more efficient and economical choice. For tasks that require advanced analytics, decision-making, and adaptive capabilities, an AI Agent may be more advantageous.
In many cases, AI Agent and RPA can complement each other and be used in combination for a more comprehensive automation solution.
Hybrid Approach: Combining RPA & AI Agents
The convergence of RPA and AI Agents is giving rise to intelligent automation ecosystems that deliver greater value than either technology could achieve independently. Imagine a financial institution’s fraud detection system: RPA bots first aggregate structured transaction data from siloed banking platforms with robotic efficiency, while AI Agents simultaneously analyze these datasets alongside unstructured information like customer behavior patterns and global fraud trends.
When anomalies surface, the AI component makes risk-weighted decisions, then triggers RPA workflows to freeze accounts or generate regulatory reports – all within milliseconds. This symbiotic relationship enables organizations to automate entire business processes rather than isolated tasks. If we are advocating for layered automation architectures where RPA handles the “muscle” of task execution, computer vision extracts insights from documents, and AI Agents provide the “brain” for strategic decision-making. Implementation typically follows a crawl-walk-run trajectory, starting with RPA pilots before progressively integrating AI components as organizational maturity grows.
Octoparse AI is working to encapsulate complex RPA processes into out-of-the-box apps, while also retaining the process building capabilities of RPA. In the near future, Octoparse AI will support the generation of runnable workflows through natural language interactions with AI Agents. This will be an organic and great combination.
Conclusion: The Evolution of Automation
The automation landscape is rapidly evolving toward cognitive-first architectures. By 2025, over 90% of RPA implementations are projected to incorporate AI capabilities as standard features, transforming basic bots into self-optimizing systems. We’re already seeing early examples of AI Agents that dynamically rewrite RPA scripts based on process mining insights or automatically adjust inventory algorithms in response to geopolitical events. Emerging technologies like small language models (SLMs) will enable domain-specific AI Agents that understand industry jargon and compliance nuances, while advances in multimodal AI will allow systems to process video, voice, and text in unified workflows.
Perhaps most significantly, automation platforms are evolving into self-assembling ecosystems – picture an AI project manager that autonomously coordinates teams of RPA bots, AI diagnostic tools, and human specialists based on real-time business priorities. For enterprises, this underscores the need to build adaptable automation strategies that leverage both technologies’ complementary strengths while preparing infrastructure for AI-native workflows.
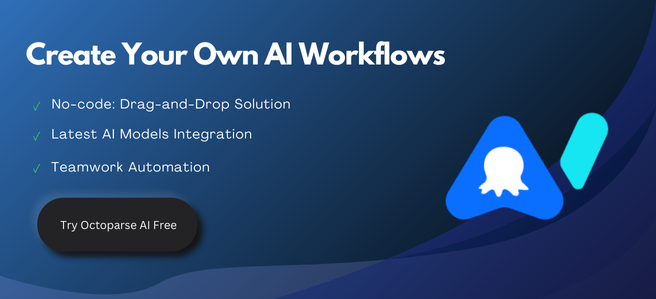